Data is the lifeblood of modern organizations, and ensuring its accuracy, consistency, and integrity is crucial for making informed decisions and driving business success. As businesses deal with vast amounts of data, two common approaches for improving data quality are master data cleansing and data quality management.
While they share similarities, they also have distinct differences. In this blog post, we will explore and compare master data cleansing and data quality management, highlighting the key features, processes, and benefits of these two strategies, and how they can work together to ensure reliable data for your business.
Understanding Master Data Cleansing :
Master data cleansing refers to the process of identifying and correcting errors, inconsistencies, and redundancies in master data. Master data includes critical business information such as customer data, product data, and supplier data, which are used as reference data across different systems and applications.
Common data quality issues in master data include duplicate data, inconsistent formatting, and outdated information. To address these issues, various techniques and strategies can be employed, such as data profiling to assess data quality, data matching to identify and merge duplicate data, and data enrichment to enhance data with additional information.
Key Features:
- Focuses on specific master data domains.
- Typically carried out as a one-time or periodic process.
- Involves data validation, standardization, enrichment, and deduplication in specific master data sets.
- Usually carried out by data experts or data stewards with domain-specific knowledge.
Benefits:
- Improves the accuracy and consistency of data in specific master data domains.
- Enhances data quality in key areas of the organization, such as customer relationship management (CRM), supply chain management, and financial management.
- Reduces the risk of errors, duplicates, and inconsistencies in master data, which can lead to costly mistakes and business disruptions.
- Enhances data-driven decision-making by providing reliable and trustworthy master data for analysis and reporting.
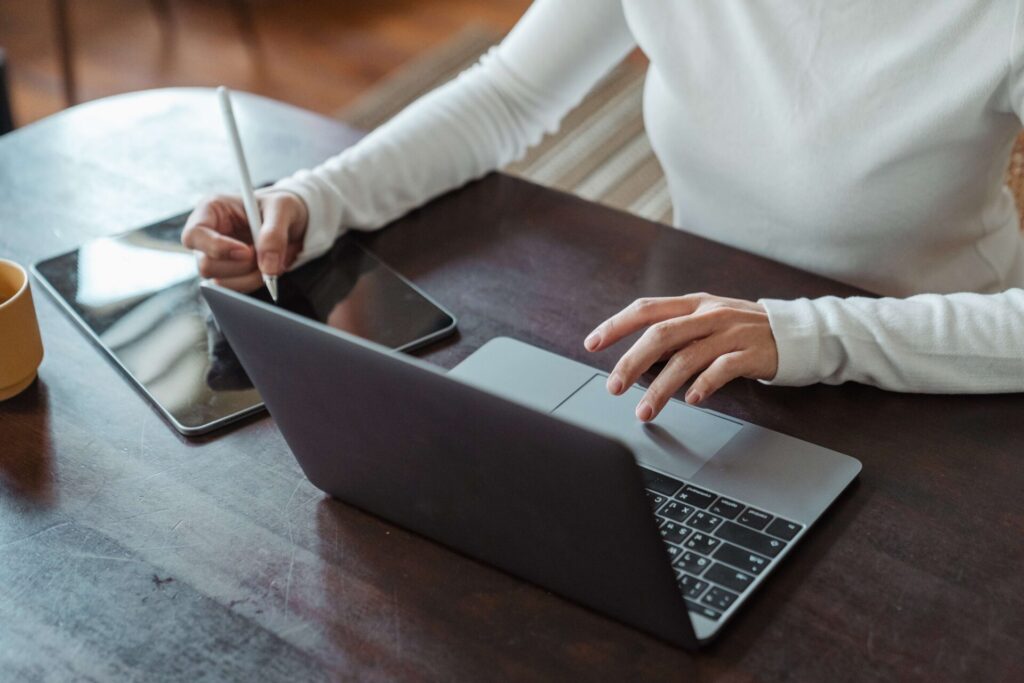
Understanding Data Quality Management:
Data quality management, on the other hand, encompasses a broader approach to ensure data quality throughout the data lifecycle. It involves the establishment of data quality standards, policies, and processes, and the implementation of data quality tools and technologies.
Key principles and best practices of data quality management include data validation to ensure data meets predefined criteria, data standardization to ensure consistent data formatting, and data governance to establish data ownership and accountability.
Data quality tools and technologies, such as data quality software, frameworks, and metrics, can aid in the identification, measurement, and improvement of data quality. The advantages of effective data quality management include improved decision-making, enhanced customer satisfaction, and increased operational efficiency.
Key Features:
- Encompasses a comprehensive approach for overall data quality improvement.
- Covers all types of data, including master data, transactional data, and reference data.
- Involves data profiling, data validation, data standardization, data enrichment, data monitoring, and data governance processes.
- Includes data quality assessment, measurement, and reporting to ensure continuous improvement.
- Requires involvement from various stakeholders, including data owners, data stewards, IT, and business users.
- This may involve implementing data quality tools or technologies for automation and scalability.
Benefits:
- Improves data quality across the entire organization, ensuring consistency and integrity in all data used for decision-making.
- Establishes data quality as an ongoing process, enabling continuous improvement and monitoring of data quality standards.
- Reduces the risk of errors, inconsistencies, and duplicates in all types of data, minimizing the potential impact on business operations.
- Facilitates compliance with data regulations and industry standards by ensuring data accuracy, consistency, and integrity.
- Enhances trust in data for strategic planning, operational efficiency, and customer satisfaction.
Master Data Cleansing vs. Data Quality Management:
While both master data cleansing and data quality management are aimed at ensuring data quality, they have some differences in their scope and focus.
- Master data cleansing primarily focuses on identifying and correcting errors in master data, while data quality management encompasses a broader approach that includes data validation, standardization, governance, and overall data quality improvement.
- Master data cleansing is typically applied to specific master data sets, such as customer or product data, whereas data quality management is a more comprehensive approach that covers data across the entire organization.
However, it’s important to note that master data cleansing and data quality management can work together synergistically.
- For example, master data cleansing can be considered a part of data quality management efforts, ensuring that master data is accurate and reliable.
- Conversely, data quality management can provide the framework and processes for identifying and addressing data quality issues, including master data.
- By combining both approaches, businesses can establish a holistic approach to ensure reliable data for their operations.
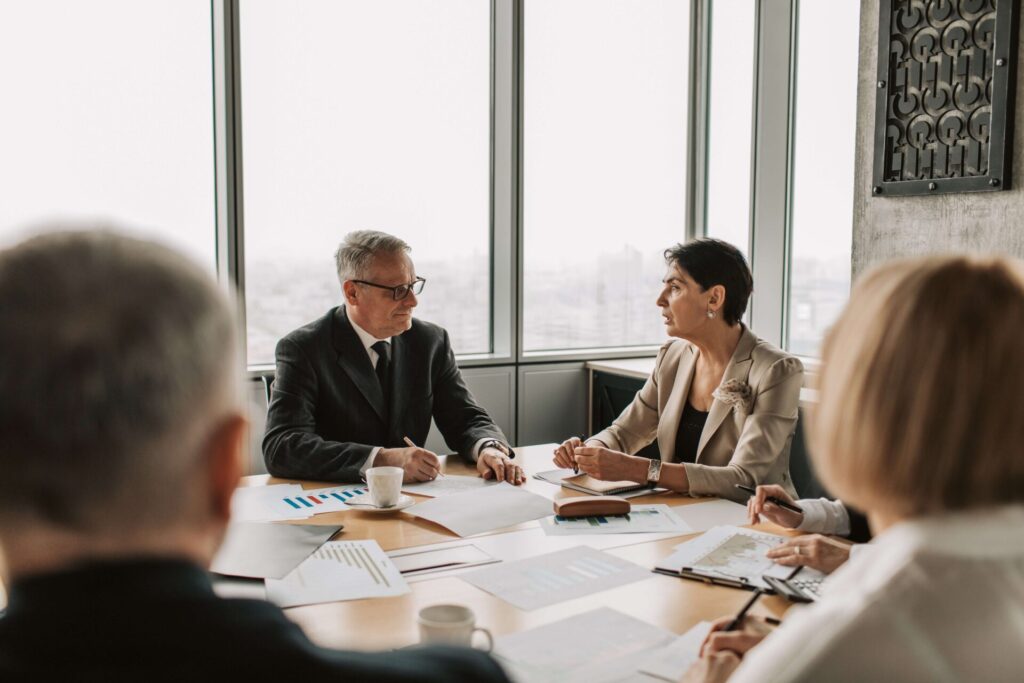
Challenges and Considerations
Implementing master data cleansing and data quality management practices can come with challenges. Some common challenges include the complexity of data sources, data integration across different systems, and the need for data governance and ownership.
Organizations may also face challenges in identifying and correcting data quality issues promptly and maintaining data quality consistently over time. It’s important to consider these challenges and develop strategies to overcome them, such as establishing data quality standards, implementing data quality tools, and promoting data governance practices.
Final Thoughts:
In today’s competitive business landscape, reliable data is a strategic asset that can drive business success. Ensuring data quality through master data cleansing and data quality management is essential for organizations to gain a competitive edge, improve operational efficiency, and maintain customer satisfaction.
By combining the strengths of both approaches and overcoming challenges, businesses can establish a solid foundation for data-driven decision-making and achieve their business goals.
In conclusion, master data cleansing and data quality management are critical strategies for ensuring reliable data in organizations. While they have differences in scope and focus, they can work together to establish a comprehensive data quality framework.
By addressing challenges and implementing best practices, businesses can ensure the accuracy, integrity, and consistency of their data, leading to improved decision-making, operational efficiency, and customer satisfaction.
Invest in data quality management and master data cleansing to ensure your business’s data is trustworthy and reliable, giving you a competitive advantage in the market.
We hope you found this blog post informative and useful in understanding the importance of master data cleansing and data quality management for your business. For further guidance and assistance in implementing these strategies in your organization, feel free to reach out to our experts at info@codasol.com
Reliable data is the foundation of successful business operations, and investing in data quality management and master data cleansing is a wise decision to ensure your data is accurate, consistent, and reliable. Stay ahead of the competition with reliable data and make informed decisions for your business’s success.
5 reasons why you need Prosol to simplify your Business
Integration
With Prosol, you can get a Master Data Curation Workflow process embedded within your core master data management application.
Cloud-based tool
Our real-time Master Data Management allows you to intelligently govern and synchronize materials data across multiple applications and systems. It ensures that data quality does not decay and remains intact all the time.
Effective search engine
CodaSol has developed Machine Learning Catalogs from the vast datasets we have gathered for years in the form of ProPedia based on Industry Standard Classifications like UNSPSC. Prosol uses this to identify duplicate product codes and eradicate them quickly.
Customizable features
Prosol provides a custom template engine that can help you generate Material codes that adhere to your policies. Prosol also allows multiple templates to be used for different material categories as well.
Global standard
By Leveraging Prosol, You can ensure that new item descriptions, modifications, or deletions are always in sync with global standards.
Bonus advantages:
- Prosol helps in Procurement Optimization by avoiding intentional and unintentional excessive purchases.
- It has a Secured data repository.