Data Quality :
Data quality ensures data in an organization is reliable, consistent, complete, and accessible for planning, decision-making, and identifying whether it is fit to make massive changes. It measures data based on redundancies, data silos, duplication, inconsistencies, and validity.
Data quality is crucial because the accumulation of data is a waste of time if we are not going to use it. It should contain reliable and trustworthy data to make other decisions based on that. It is possible only by constantly checking whether the added data is accurate.
Data Integrity :
Data integrity goes beyond data quality. Data integrity ensures the quality of data can be trusted or not. It digs deeper into the context of the origin of the data by scrutinizing the entered data based on reliable sources and maintains consistency with updates and changes in the future.
Data integrity tackles data corruption and examines other pillars like data security, enrichment, and data validity.
Data Quality vs. Data Integrity :
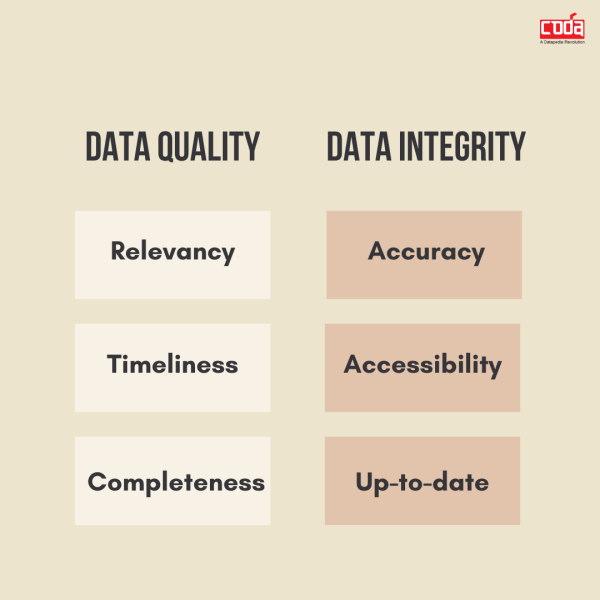
From the above definitions, it is clear that both data quality and data integrity play vital roles in completing data and making it accessible for everyone to move an organization further with better decisions and strategies. It is in Organization’s place to know the difference between them and implement them accordingly.
Data integrity is structured and strategic compared to data quality. Data integrity is one of the pillars of ensuring overall data quality. So having quality data will make the process of ensuring data integrity easier.
To conclude, the elaborative data integrity process is minimized by focusing on the initial stages of acquiring quality data. To maintain quality data, an organization can ensure they are free of data quality issues and depend on software like Prosol to have an effective, consistent, and reliable data system.