Master data cleansing is a crucial process for businesses that rely on accurate and reliable data to make informed decisions. However, it is not without its challenges. Duplicate records, inconsistencies, and missing data are just a few of the issues that can arise during the master data cleansing process.
In this blog post, we will explore some of the best practices, tools, and trends in master data cleansing to help organizations improve their data quality and make better business decisions.
Common Challenges Faced During Master Data Cleansing
Master data cleansing is a complex and time-consuming process that requires careful planning and execution. Some of the most common challenges faced during this process include:
- Duplicate records: Duplicate records can confuse and lead to inaccurate data analysis. It is important to identify and eliminate duplicates during the master data cleansing process.
- Inconsistencies: Inconsistent data can lead to incorrect insights and hinder decision-making. It is important to standardize data and ensure consistency across all sources.
- Missing data: Missing data can create gaps in knowledge and hinder data analysis. It is important to identify and fill in missing data to ensure a complete and accurate picture.
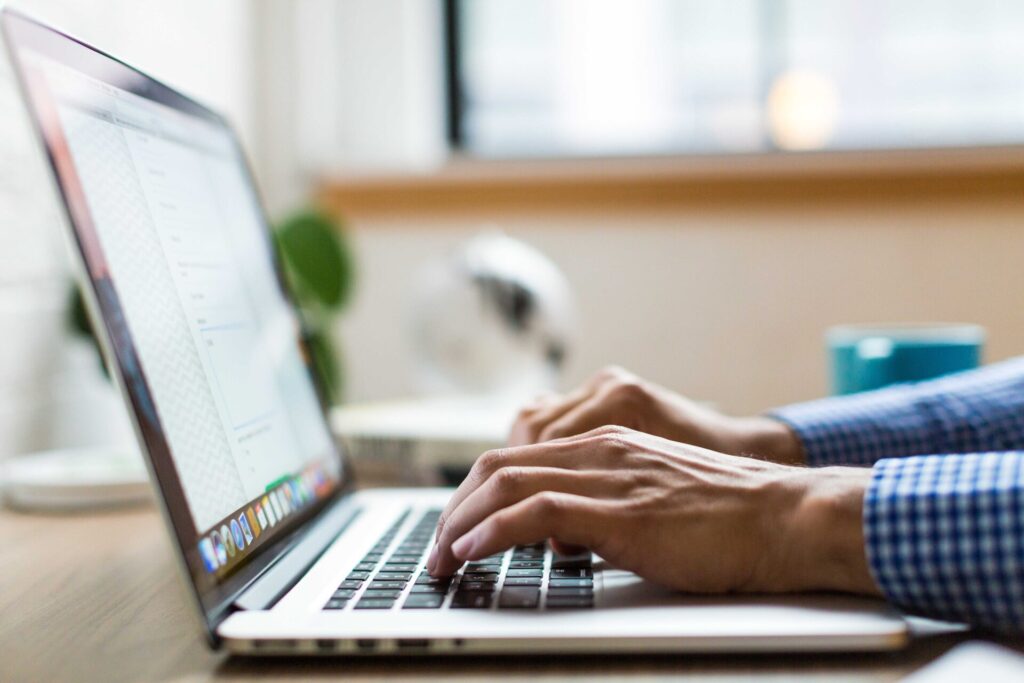
Best Practices for Successful Master Data Cleansing
To ensure a successful master data cleansing process, organizations should follow these best practices:
- Establish clear goals and metrics: Before starting the master data cleansing process, it is important to establish clear goals and metrics to measure success. This will help keep the project focused and ensure that it is aligned with business objectives.
- Involve stakeholders: Master data cleansing affects multiple areas of the business, so it is important to involve all relevant stakeholders in the process. This includes IT, business analysts, data scientists, and end-users.
- Implement quality controls: Quality controls such as data profiling and data validation can help ensure that the master data is accurate and consistent. These controls should be implemented throughout the entire master data cleansing process.
Benefits of Master Data Cleansing
Implementing a master data cleansing process can provide many benefits to organizations, including:
- Improved data accuracy: By identifying and resolving data quality issues, organizations can improve the accuracy of their data and reduce errors in analysis.
- Increased operational efficiency: With accurate and consistent data, organizations can streamline their processes and reduce the time and resources needed for data analysis.
- Better decision-making: By having access to reliable and consistent data, organizations can make more informed decisions and achieve better business outcomes.
Tools and Technologies for Master Data Cleansing
Fortunately, there are many tools and technologies available in the market to help organizations achieve better data quality.
At Codasol, we have developed Prosol, a powerful data cleansing tool that can help organizations achieve better data quality. Prosol is designed to address the most common data quality issues, such as incorrect, incomplete, or inconsistent data.
One of the unique features of Prosol is its ability to automatically identify and correct errors in large data sets. The tool uses advanced algorithms to detect and fix data errors, which can help organizations save time and improve data accuracy.
Prosol also offers a range of data profiling and analysis tools that can help organizations understand the quality of their data and identify areas for improvement. For example, the tool can identify duplicate records, missing data, or inconsistent data values, which can help organizations take proactive steps to improve their data quality.
But Prosol is not just about technology. We understand that data cleansing is a complex process that requires human expertise and intervention. That’s why we offer a range of consulting services to help organizations develop a data-cleansing strategy that aligns with their business goals.
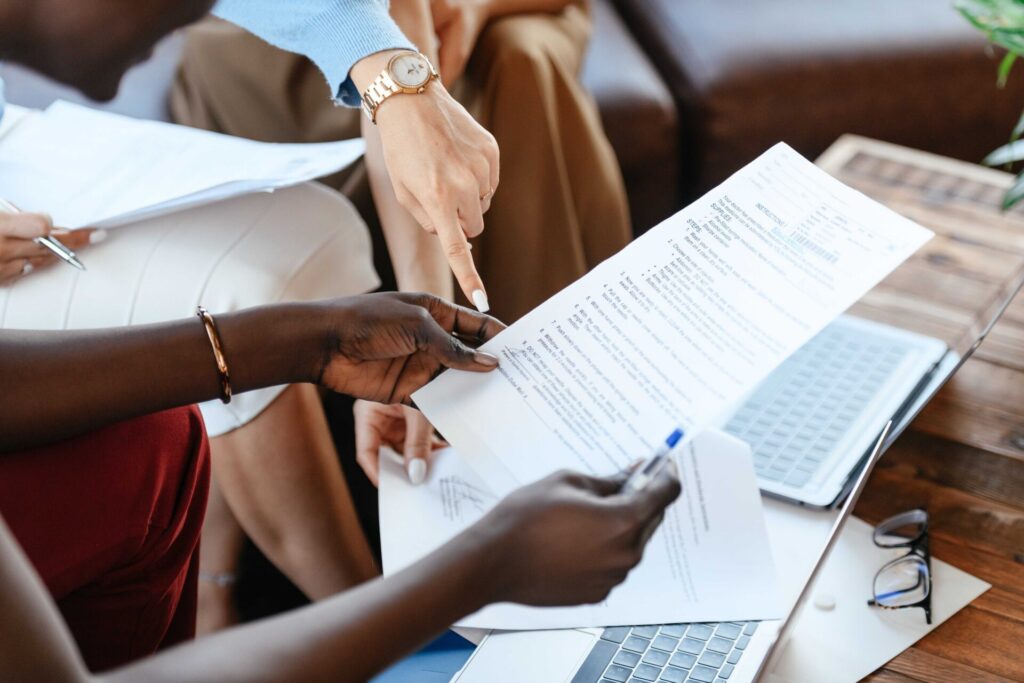
Our team of experienced data analysts and consultants can help organizations define their data quality goals, develop data cleansing processes, and implement best practices for maintaining high-quality data. We believe that the key to successful data cleansing is a combination of technology and human expertise, and we strive to deliver both through our Prosol tool and consulting services.
If you’re interested in learning more about Prosol and how it can help improve your data quality, we encourage you to visit our website at https://codasol.com/prosol. We also offer a free demo of the tool, so you can see it in action for yourself.
Master Data Cleansing Trends and Future Directions
Master data cleansing is an evolving field, and new trends and technologies are emerging all the time. Here are a few of the most promising trends to keep an eye on:
- Artificial Intelligence and Machine Learning: Artificial intelligence and machine learning are becoming increasingly important in the master data cleansing process. These technologies can automate many of the repetitive tasks involved in data cleansing, such as identifying and removing duplicate records or filling in missing data. They can also help identify patterns and correlations in the data that might not be immediately apparent to human analysts.
- Data Governance: Another important trend in master data cleansing is the growing importance of data governance. Data governance refers to the policies, procedures, and technologies that organizations use to manage their data assets. By establishing clear data governance policies, organizations can ensure that their master data cleansing process is consistent and effective across all business units and data sources.
- Blockchain technology: Blockchain technology can be used to create a secure and transparent master data management system, ensuring data privacy and security.
- Cloud-based solutions: Cloud-based solutions offer scalability, flexibility, and cost-effectiveness, making them a popular choice for master data cleansing.
The potential impact of these trends on the future of master data cleansing is significant. AI and machine learning, for example, could drastically reduce the time and resources needed for data cleansing, while improving accuracy and consistency. Data governance, blockchain technology, and cloud-based solutions, on the other hand, could help ensure that the data cleansing process is aligned with business objectives, privacy, security, and compliance requirements.
Master Data Cleansing and Compliance
Compliance with regulatory requirements is a critical concern for organizations across all industries. Failure to comply with regulations such as GDPR, CCPA, and HIPAA can result in significant financial penalties and reputational damage. Master data cleansing plays an essential role in meeting these compliance requirements.
For example, GDPR requires organizations to ensure that their data is accurate and up-to-date. By implementing a robust master data cleansing process, organizations can ensure that they are complying with this requirement. Similarly, HIPAA requires organizations to ensure that their data is secure and protected from unauthorized access. By using secure data cleansing tools and technologies, organizations can ensure that their data is protected from security breaches and other threats.
Master data cleansing can also improve organizations’ overall data privacy and security. By implementing data cleansing best practices and using the right tools and technologies, they can ensure that their data is accurate, consistent, and secure.
Final Thoughts :
Master data cleansing is a complex process that requires careful planning, execution, and ongoing maintenance. By following best practices, using the right tools and technologies, and staying up-to-date on emerging trends and regulatory requirements, organizations can improve their data quality, comply with regulatory requirements, and make better business decisions.
The future of master data cleansing looks promising, with emerging technologies such as AI and machine learning and a growing emphasis on data governance expected to play a significant role in the years to come.