Data is the backbone of every business in this digital age. But what happens when that data is filled with duplicates, inconsistencies, and errors? It leads to inefficiencies, compliance risks, and poor decision-making, all of which can cost businesses millions.This is where data cleansing and standardization come in, helping organizations detect errors, ensure accuracy and consistency, and enable seamless integration across systems. In this blog, we’ll explore the top 10 best practices for effective data cleansing and standardization.
What is Data Cleansing?
Data cleansing, the first initiative for effective data management, is the process of detecting, removing, and correcting duplicate, inconsistent, and incomplete data from the dataset. Also, fixing typographical errors, misspellings, and incorrect entries to ensure top-notch data quality within the enterprises for seamless data integration and flawless data management.
What is Data Standardization?
Data standardization is the process of converting unstructured, incomplete, and inconsistent data, generated from various resources, to fit into a uniform, predefined format to create consistent naming conventions, formats, and structures. It helps the organization with data integration, analysis, and operational efficiency across departments.
How Data Cleansing and Data Standardization Uplift Data Management
Data Accuracy
The cleansed and standardized data frees up the data set by eliminating inaccurate, duplicate, redundant information and other hidden or dark data. This streamlines the organization to achieve high-quality data while meeting industry-specific regulatory compliance.
Improve Data Consistency
Data standardization ensures that data follows a uniform structure, format, and naming convention across different platforms and departments. This consistency prevents data mismatches between systems such as ERP and CRM, making it easier to integrate, retrieve, and analyze information.
Regulatory Compliance
Standardized data helps ensure compliance by maintaining consistent formats and accurate data, reducing risks like breaches, legal penalties, and reputational damage. For example, in healthcare, standardizing patient data to meet HIPAA guidelines safeguards confidentiality and ensures regulatory compliance.
Reduced Cost
With a cleaner and standardized dataset, organizations can optimize storage, reducing costs associated with managing and storing redundant or irrelevant data. Additionally, streamlined data processes minimize the need for manual data cleaning and integration, further lowering operational expenses.
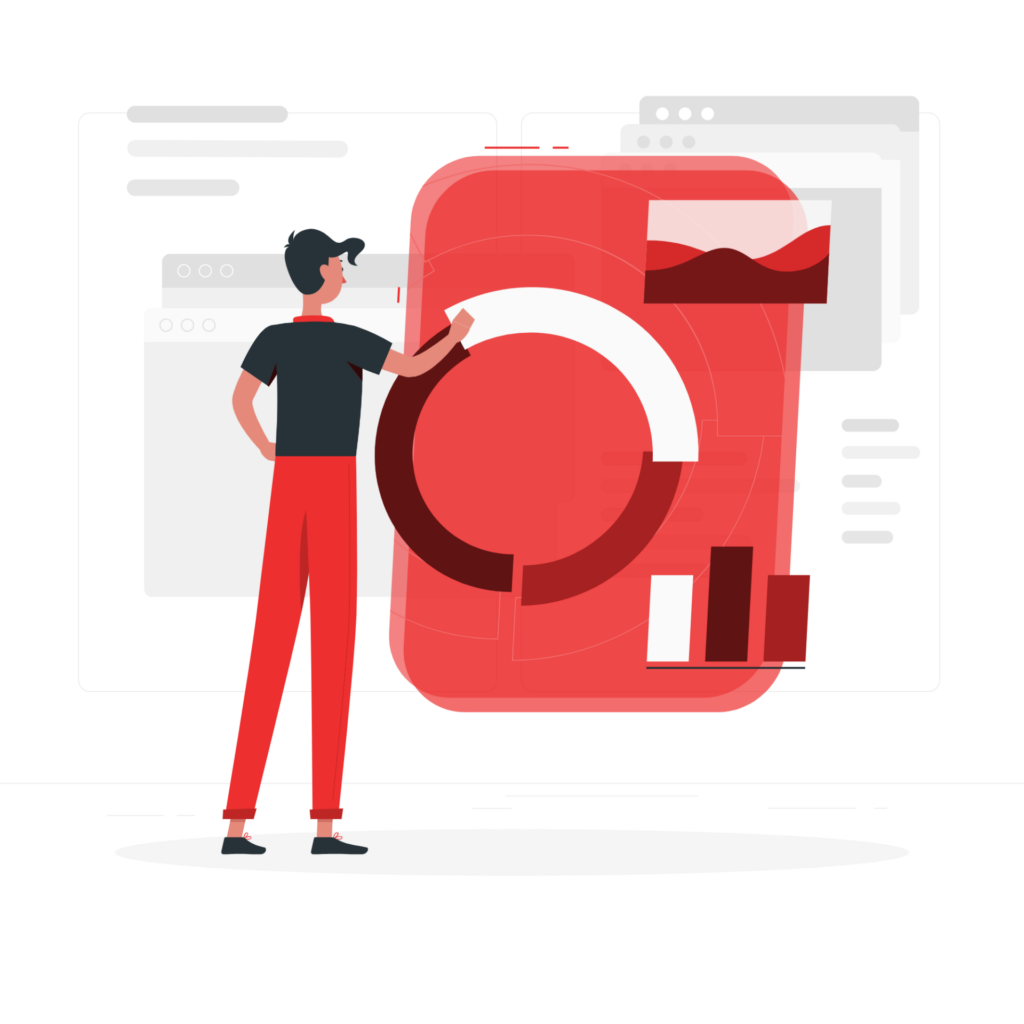
Don’t let poor data management affect your business decisions.
Top 10 Best Practices for Data Cleansing and Standardization
1. Understand the source and nature of your data.
Before applying any data cleansing or standardization processes, profile your data. Understand its origins, formats, discrepancies, and any gaps. Utilize data quality profiling tools to identify and assess the accurate, complete, and consistent data across systems. Conduct a periodic data audit to get rid of data quality issues.
2. Define Clear Data Standards
Establish a standard data format that can be accessible widely in an enterprise to ensure data consistency. These standards should specify acceptable formats, naming conventions, and permissible values for key data attributes.
3. Removing Duplicate Data
Multiple copies of the same data will skew data analysis and often lead to double counting of data. Data deduplication and fuzzy data matching are effective ways to streamline operations.
4. Validate Data at Entry Points
Data validation is the best way to prevent anomalies and bad data from the entry level. Implement validation rules for mandatory fields, format compliance, and other essential areas to reduce errors and data legal issues.
5. Utilize Data Enrichment Techniques
Data enrichment, the process of adding additional data value points sourced from both the internal and external vertices for data enhancement, is the common technique practiced in the data management organization. It not only improves data quality but also enhances its usability for analytics and decision-making.
6. Monitor Data Quality Metrics
Regular tracking of key performance indicators (KPIs) related to data quality, such as accuracy, completeness, and timeliness. Set benchmarks and monitor progress over time to ensure continuous improvement.
7. Automate Data Cleansing Workflows in Data Cleansing and Standardization
Use automated data cleansing tools to reduce manual errors, workflow complexities, and data processing time. Codasol’s AI/ML-driven data cleansing tool Prosol offers advanced workflows, identifies duplicate records, supports data visualization and automates material codes to save time and costs.
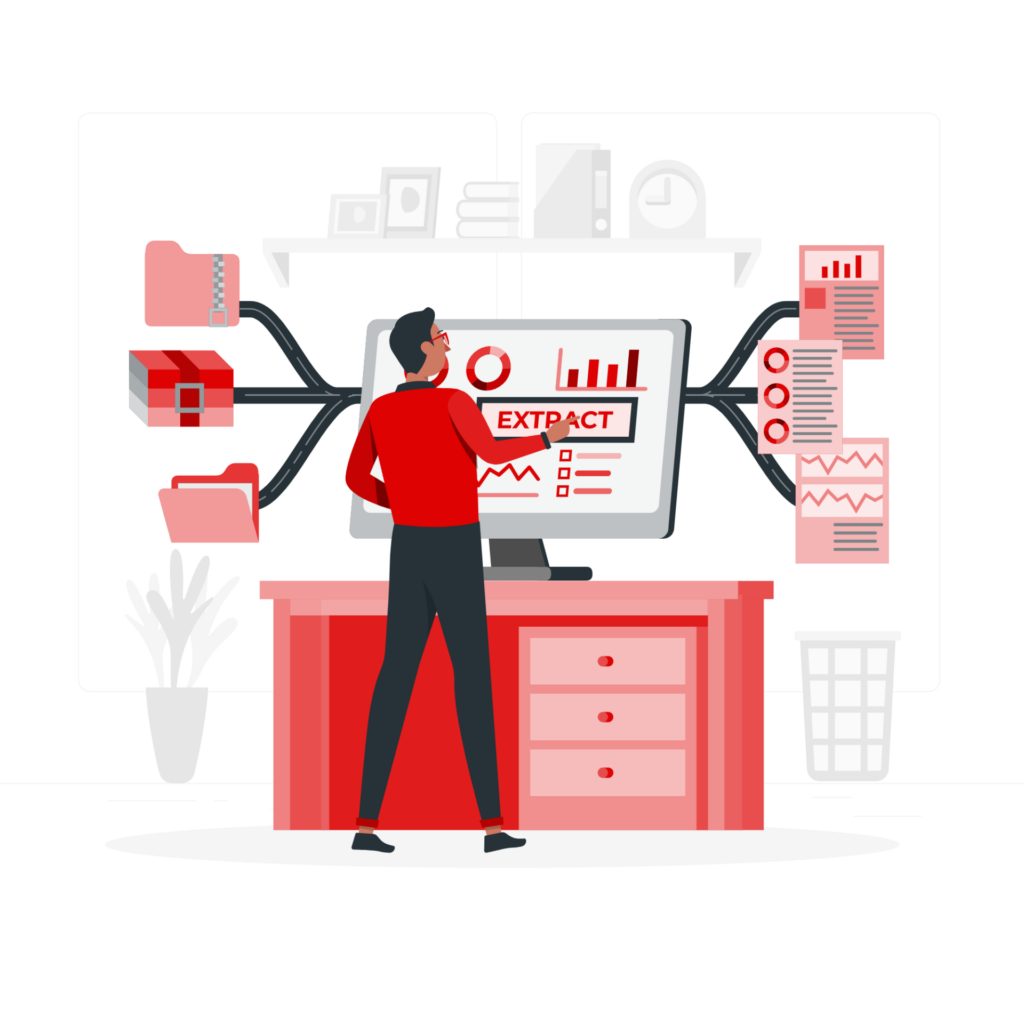
Struggling with inconsistent or duplicated data? It’s time to take action.
8. Implement Data Governance
Data governance: the unavoidable approach makes MDM stand out from any illegal penalties. By establishing a clear governance framework that defines roles, responsibilities, and processes to maintain data integrity. It also assigns data stewards to have transparency to stakeholders for the entire cleansing and standardization efforts.
9. Invest in Continuous Training for Data Cleansing and Standardization
Technology alone doesn’t solve all data quality issues it’s also in the hands of employees to make healthy data across the enterprise. Frequent or continuous education about data quality, standards, and processes makes them aware of data management.
10. Conduct Regular Data Audits
Regular audits help identify any emerging data quality issues and hidden data inconsistencies and ensure that data processes remain aligned with business goals. This proactive approach minimizes risks, ensures compliance, and maintains high-quality data over time.
Challenges in Implementing Data Cleansing and Standardization
Data Discovery and Profiling
In some cases, it is pretty hard to understand the data structure, format, values, and other mandatory details from the large dataset. As businesses shift to cloud storage, managing and integrating data from multiple sources with different structures and formats becomes increasingly complex. Implementing robust data profiling is essential to identify inconsistencies, redundancies, and gaps before standardization can begin.
Addressing Data Quality in Data Cleansing and Standardization
Another challenging task before implementing data standardization is to identify the data quality gaps, like where your data lacks in accuracy, completeness, timeliness, and accessibility. And spotting the missing key data and counts of duplicate data.
Governance and Accountability
Lack of clear ownership, like roles and responsibilities, and accountability for data quality leads to repeated errors and inconsistencies. Making cleansing and standardization intensive. Setting clear guidelines for data quality and governance frameworks to resolve costly mistakes and prevent operational inefficiencies.
Challenges with Legacy Systems
Old legacy systems lack the flexibility and scalability to adopt with advanced data cleansing and standardization. These hinder data synchronization. Also, upgrading new legacy systems results in increased cost and time.
How Coda Simplifies Data Cleansing and Standardization
Coda makes data cleansing and standardization easier and more efficient by leveraging advanced AI/ML-driven tools and automated workflows. Here’s how:
AI-Driven Data Cleansing:
Coda’s Prosol tool automatically identifies and removes duplicates, corrects errors, and addresses inconsistencies, ensuring that data is accurate and reliable across systems.
Automated Standardization:
Simplifies the process of standardizing data by converting unstructured and inconsistent data into a uniform format, making it easier to integrate, analyze, and report across different platforms and departments.
Real-Time Data Profiling:
Continuously scan and assess data quality, identifying discrepancies, redundancies, and gaps, ensuring that your data is always clean and ready for analysis.
Cost Efficiency:
Coda lowers operational costs by automating data cleansing and standardization, reducing manual corrections, and optimizing storage by eliminating redundant data.
Seamless Integration Across Systems:
Standardize data streamlines data integration, ensuring smoother data flows between different systems.
Wrapping thoughts
Data cleansing and standardization are not one-time activities; they require ongoing effort, supported by the right tools and strategies. They are business-critical strategies that drive efficiency, compliance, and smarter decision-making. Without clean, standardized data, organizations face operational inefficiencies, compliance risks, and lost opportunities.
Codasol specializes in best practices in data cleansing and standardization, which helps your organization gain accurate, consistent, and trustworthy data, paving the way for smarter decision-making and better business outcomes.
Don’t let messy data hold your business back. Get in touch with Codasol to see how our solutions can help you maintain accurate, reliable data for smarter decisions and greater business success.
Take the First Step Towards Clean, Consistent Data Today.